Hi there, we’re Harisystems
"Unlock your potential and soar to new heights with our exclusive online courses! Ignite your passion, acquire valuable skills, and embrace limitless possibilities. Don't miss out on our limited-time sale - invest in yourself today and embark on a journey of personal and professional growth. Enroll now and shape your future with knowledge that lasts a lifetime!".
For corporate trainings, projects, and real world experience reach us. We believe that education should be accessible to all, regardless of geographical location or background.
1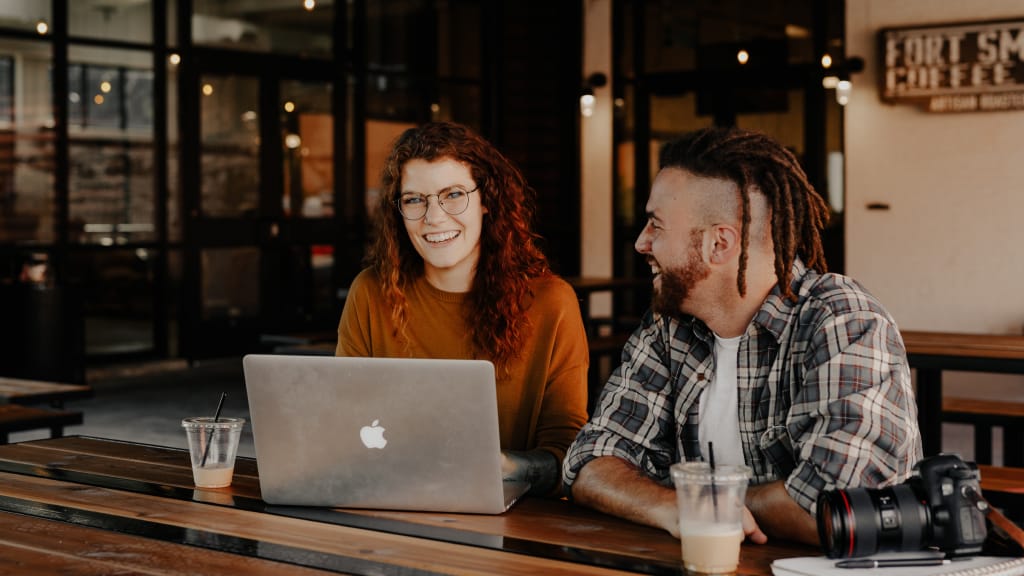
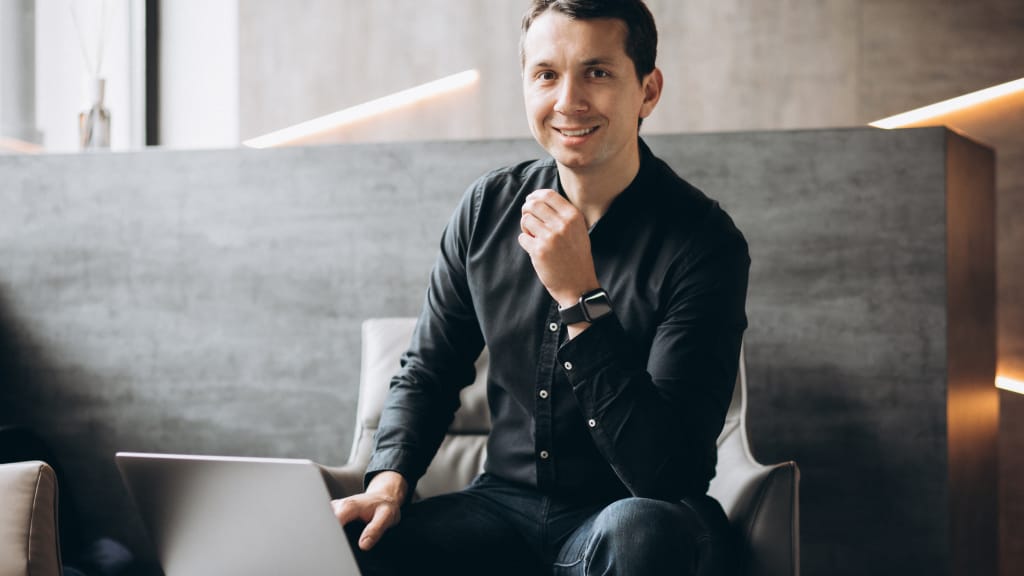
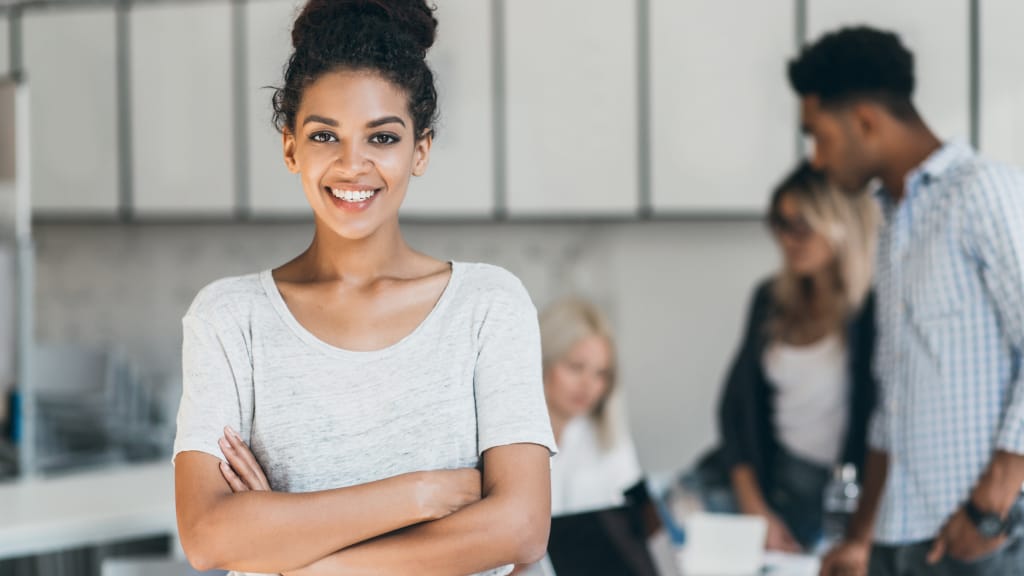
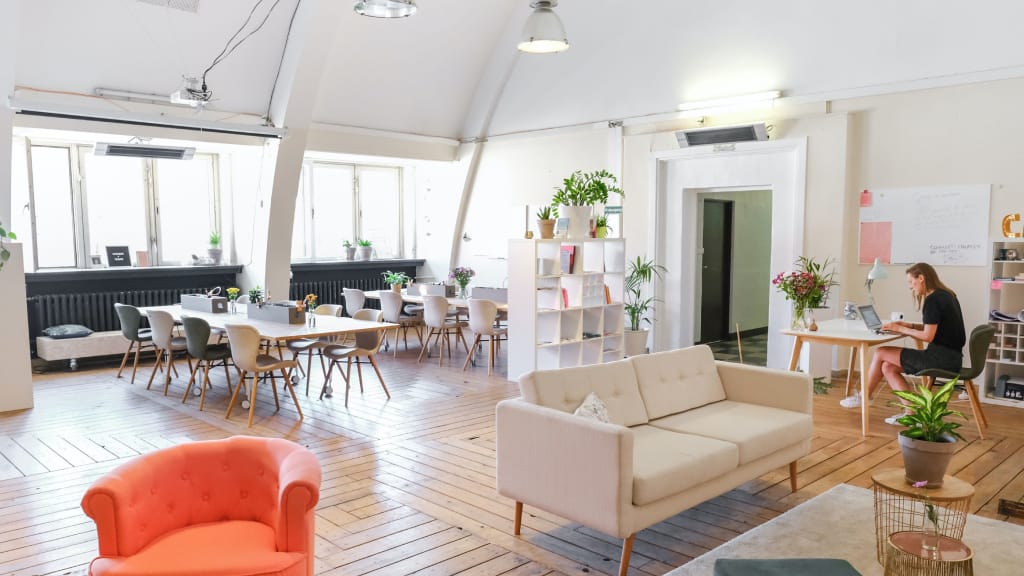
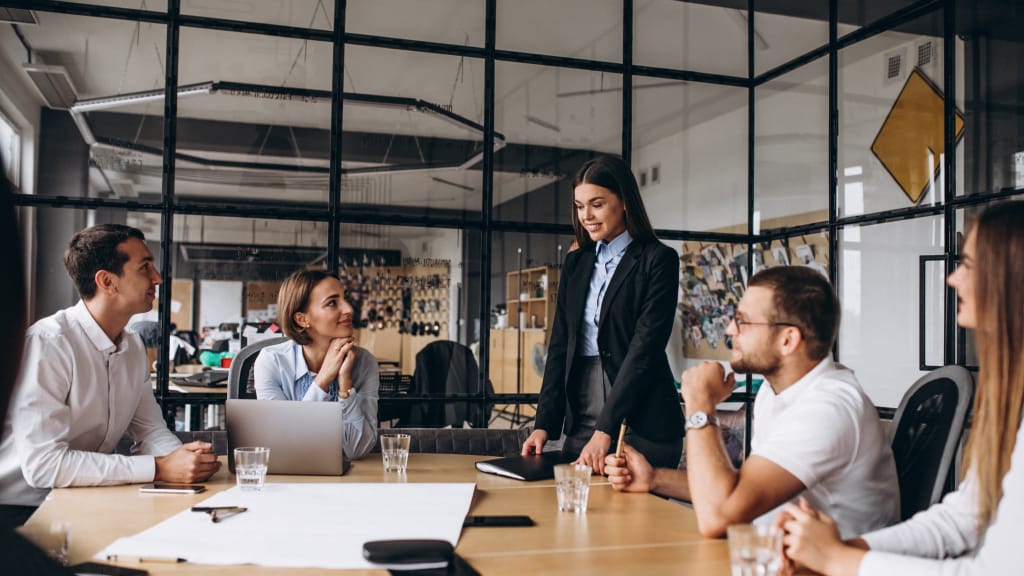
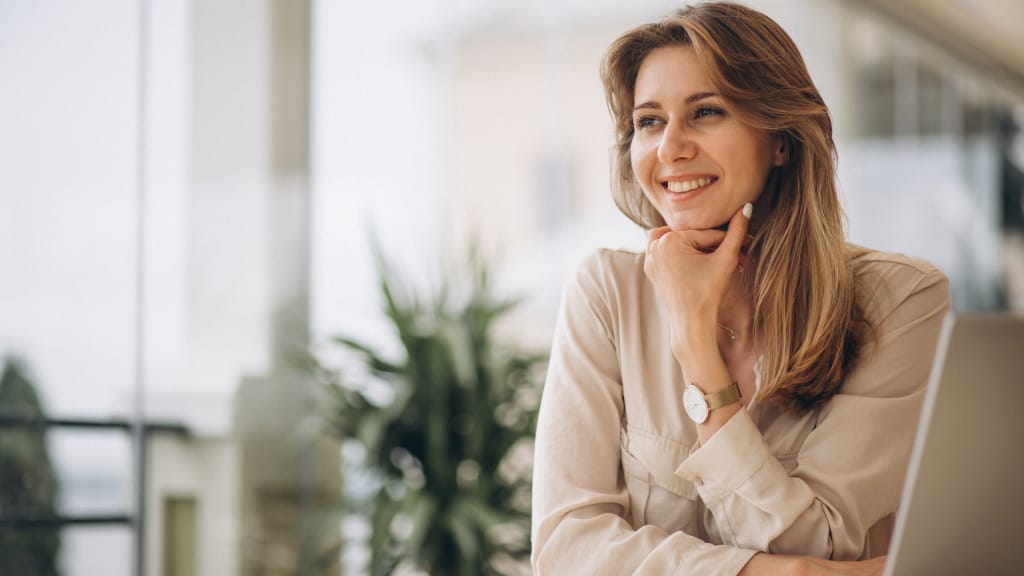
Python for Data Science
Python has emerged as one of the most popular programming languages for data science. Its simplicity, powerful libraries, and extensive ecosystem make it an ideal choice for tasks such as data manipulation, analysis, visualization, and machine learning. In this article, we'll explore how Python can be used for data science and provide example code snippets to showcase its capabilities.
Example 1: Data Manipulation with Pandas
Pandas is a widely-used Python library for data manipulation and analysis. Here's an example of using Pandas to read and analyze a CSV file:
import pandas as pd
# Read the CSV file into a Pandas DataFrame
data = pd.read_csv('data.csv')
# Display the first few rows of the DataFrame
print(data.head())
# Get summary statistics of the data
print(data.describe())
# Filter the data based on a condition
filtered_data = data[data['age'] > 30]
# Calculate the average salary of the filtered data
average_salary = filtered_data['salary'].mean()
print("Average Salary:", average_salary)
In this code, we import the pandas library and use the read_csv function to read a CSV file into a Pandas DataFrame. We then use various DataFrame methods to perform operations on the data, such as displaying the first few rows, getting summary statistics, and filtering the data based on a condition. Finally, we calculate the average salary of the filtered data using the mean method.
Example 2: Data Visualization with Matplotlib
Matplotlib is a popular Python library for data visualization. Here's an example of using Matplotlib to create a line plot:
import matplotlib.pyplot as plt
# Data for x-axis
years = [2015, 2016, 2017, 2018, 2019, 2020]
# Data for y-axis
sales = [100, 150, 200, 250, 300, 350]
# Create a line plot
plt.plot(years, sales)
# Set plot title and axis labels
plt.title('Sales over Time')
plt.xlabel('Year')
plt.ylabel('Sales')
# Display the plot
plt.show()
In this code, we import the matplotlib.pyplot module as plt. We define data for the x-axis (years) and y-axis (sales). We use the plot function to create a line plot by passing the x-axis and y-axis data. We then set the plot title and axis labels using the title, xlabel, and ylabel functions. Finally, we display the plot using the show function.
Example 3: Machine Learning with Scikit-learn
Scikit-learn is a powerful Python library for machine learning. Here's an example of using Scikit-learn to build a simple regression model:
from sklearn.linear_model import LinearRegression
from sklearn.model_selection import train_test_split
from sklearn.metrics import mean_squared_error
# Load the dataset
data = pd.read_csv('data.csv')
X = data[['feature1', 'feature2']]
y = data['target']
# Split the dataset into training and testing sets
X_train, X_test, y_train, y_test = train_test_split(X, y,
test_size=0.2, random_state=42)
# Create a linear regression model
model = LinearRegression()
# Train the model
model.fit(X_train, y_train)
# Make predictions on the test set
predictions = model.predict(X_test)
# Evaluate the model using mean squared error
mse = mean_squared_error(y_test, predictions)
print("Mean Squared Error:", mse)
In this code, we import the necessary modules from scikit-learn. We load a dataset from a CSV file into Pandas DataFrame and split it into training and testing sets. We create a linear regression model using the LinearRegression class, train the model on the training data, and make predictions on the test set. Finally, we evaluate the model's performance using the mean squared error metric.
Conclusion
Python provides a comprehensive set of tools and libraries for data science tasks, making it an excellent choice for data scientists. The examples provided in this article demonstrate how Python can be used for data manipulation, visualization, and machine learning. Whether you're just starting with data science or already have experience, Python's flexibility and rich ecosystem will empower you to perform various data-related tasks efficiently. Feel free to explore these examples further and leverage Python for your data science projects!
4.5L
Learners
20+
Instructors
50+
Courses
6.0L
Course enrollments
Future Trending Courses
When selecting, a course, Here are a few areas that are expected to be in demand in the future:.
Future Learning for all
If you’re passionate and ready to dive in, we’d love to join 1:1 classes for you. We’re committed to support our learners and professionals their development and well-being.
View Courses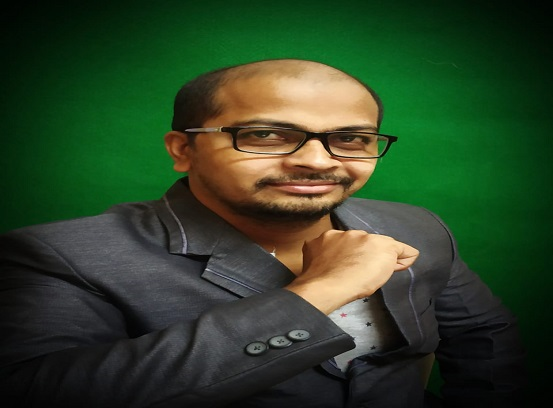
Most Popular Course topics
These are the most popular course topics among Software Courses for learners